Thursday
Oct092014
Cris Moore at SFI


Cris Moore
Optimization From Mt. Fuji to the Rockies: How computer scientists and physicists think about optimization, landscapes, phase transitions and fragile models
- We need to make qualitative distinctions between problems
- There is a Hamiltonian Path Problem—can you visit every node on a graph just once
- You can do this by a “search tree” until you end up stuck. Go back to the prior node, then begin again. This is called “exhaustive search.
- ”There is reason to believe that exhaustive search is the only way to solve such a problem
- NP (complete)
- P: polynomial-can find solutions efficiently
- NP: we can check a solution efficiently
- There is a gap between what you can check vs what you can find efficiently. This is the P vs NP problem.
- Polynomials don’t grow too badly as n grows, but NP complete, if n grows at 2^n then when n=90 it takes longer than the age of the universe to solve.
- When is there a shortcut?
- 1: divide and conquer—when there are independent sub-problems.
- 2: dynamic programming—sub-problems that are not completely dependent, but become so after we make some choices. (ie there are n nodes to the experiment, but once you take on node the next step becomes independent of the prior choices).
- 3: When greed is good—minimum spanning tree. Take the shortest edge (ie if you want to build power lines connecting cities, build the shortest connections first until a tree is built).
- Landscape (imagine a mountain range where your goal is to get to a distant highest peak) a single optimum, that we can find by climbing (no wrong way).
- Traveling salesman—big shortcuts can lead us down a primrose path.
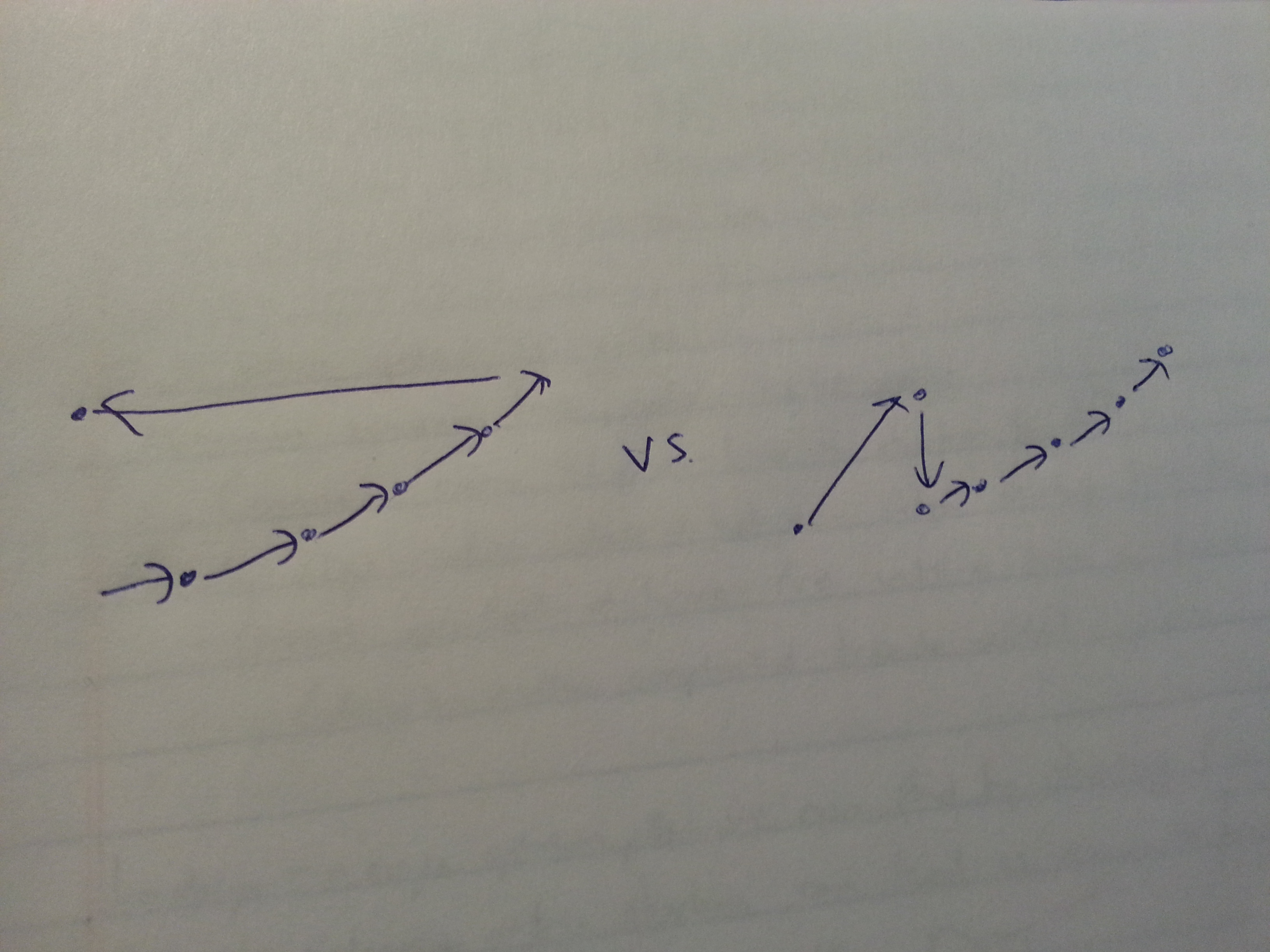
-
-
- There are many local optima where we can get stuck and it’s impossible to figure out the global optimum
-
- NP completeness=a worst case notion.
- We assume instances are designed by an adversary to encode hard problems. This is a good assumption in cryptography, but not in most of nature. We must ask: “What kind of adversary are scientists fighting against…Manichean or Augustinian?”
- The real world has structure. We can start with a “greedy tour” and make modifications until it becomes clear there is nothing more to gain.
- Optimization problems are like exploring high dimensional jewelry (multi-faceted)
- Simplex—crawls the edges quickly. Takes exponential amounts of time in the worst case, but is efficient in practice.
- 1: Add noise to the problem and the number of facets goes down
- 2: “Probably approximately correct”—not looking for the best solution, just a really good one
- Landscapes are not as dumpy as they could be. Good solutions are close to the optimum, but we might not find THE optimum. If your data has clusters, any good cluster should be close to the best.
- There are phase transitions in NP Complete (what are called tipping points)
- 3: “Sat(isfiability)”—n variables that can be true or false and a formula of constraints with three variables each. With n variables, 2^n possibilities. We can search, see if it works.
- What if constraints are chosen randomly instead of by an adversary? When the density of constraints is too high, we can no longer satisfy them all at once.
- There is a point of transition from unsolvability to solvability
- The hardest problems to solve are in the transition. When a problem is no longer solvable, have to search all options to figure that out.
- Easy, hard, frozen—the structure gets more fragile the closer you get to the solution.
- The hardest problems to solve are in the transition. When a problem is no longer solvable, have to search all options to figure that out.
- Big data and fragile problems:
- Finding patterns (inference)
- You actually don’t want the “best” model, as the “best” gives a better fit, but is subject to overfitting and thus does worse with generalizations about the future.
- Finding communities in networks (social media) is an optimization problem. You can divide into two groups to minimize the energy, but there can be many seemingly sensible divisions with nothing in common.
- You don’t want the “best” structure, you want the consensus of many good solutions. This is often better than the “best” single solution.
- If there is no consensus then there is probably nothing there at all.
- The Game of Go: unlike chess, humans remain better at Go than algos (this is true of bridge too).
- There are simply too many possible options in Go for the traditional approach with explores the entire game tree (as is done in chess).
- To win, an algo has to assume a player plays randomly beyond the prediction horizon and recomputed the probability of winning a game with each move.
- This incentivizes (and rewards the algo) for making moves that lead to the most possible winning options, rather than a narrow path which does in fact lead to victory.
- The goal then becomes broadening the tree as much as possible, and giving the algo player optionality.
- “Want to evolve evolvability” and not just judge a position, but give mobility (optionality). This is a heuristic in order to gain viability.